Learning to Solve Inverse Problems in Imaging
Rebecca Willett / University of Chicago
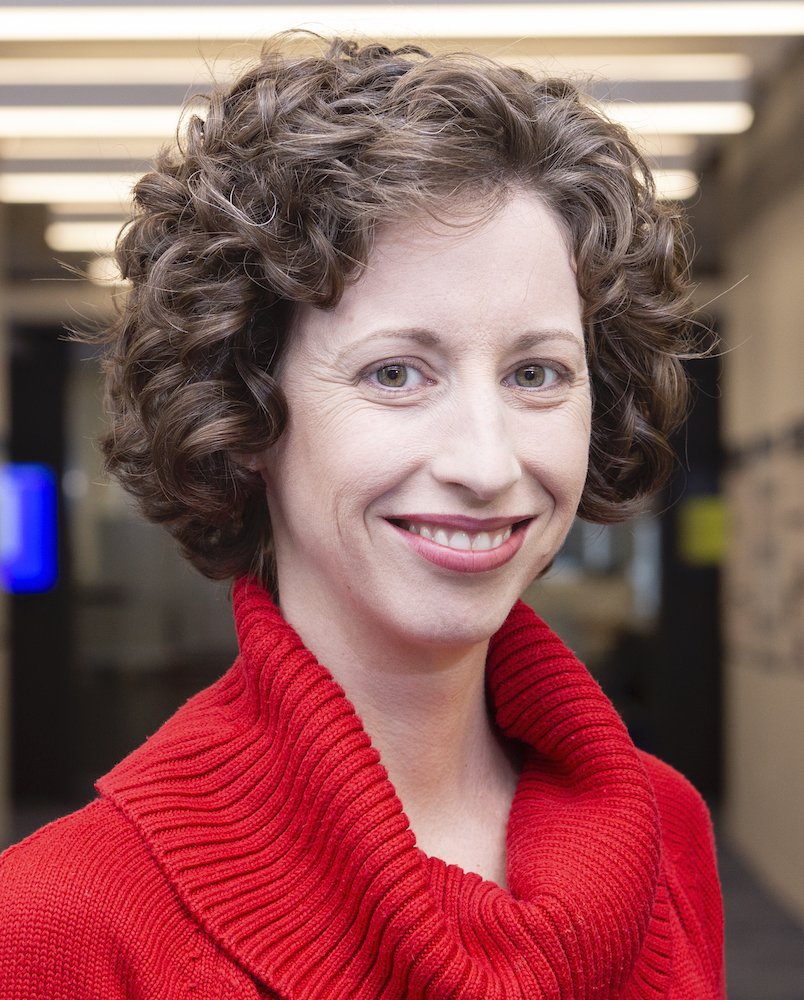
Abstract:
Many challenging image processing tasks can be described by an ill-posed linear inverse problem: deblurring, deconvolution, inpainting, compressed sensing, and superresolution all lie in this framework. Traditional inverse problem solvers minimize a cost function consisting of a data-fit term, which measures how well an image matches the observations, and a regularizer, which reflects prior knowledge and promotes images with desirable properties like smoothness. Recent advances in machine learning and image processing have illustrated that it is often possible to learn a regularizer from training data that can outperform more traditional regularizers. However, some popular approaches are highly suboptimal in terms of sample complexity, which we can see from the perspective of conditional density estimation. I will describe an end-to-end, data-driven method of solving inverse problems inspired by the Neumann series, called a Neumann network. The Neumann network architecture outperforms traditional inverse problem solution methods, model-free deep learning approaches and state-of-the-art unrolled iterative methods on standard datasets. Finally, when the images belong to a union of subspaces and under appropriate assumptions on the forward model, we prove there exists a Neumann network configuration that well-approximates the optimal oracle estimator for the inverse problem and demonstrate empirically that the trained Neumann network has the form predicted by theory. This is joint work with Davis Gilton and Greg Ongie.
Bio: Rebecca Willett is a Professor of Statistics and Computer Science at the University of Chicago. Her research is focused on machine learning, signal processing, and large-scale data science. She completed her PhD in Electrical and Computer Engineering at Rice University in 2005 and was an Assistant then tenured Associate Professor of Electrical and Computer Engineering at Duke University from 2005 to 2013. She was an Associate Professor of Electrical and Computer Engineering, Harvey D. Spangler Faculty Scholar, and Fellow of the Wisconsin Institutes for Discovery at the University of Wisconsin-Madison from 2013 to 2018. Willett received the National Science Foundation CAREER Award in 2007, was a member of the DARPA Computer Science Study Group, and received an Air Force Office of Scientific Research Young Investigator Program award in 2010.
Bio: Rebecca Willett is a Professor of Statistics and Computer Science at the University of Chicago. Her research is focused on machine learning, signal processing, and large-scale data science. She completed her PhD in Electrical and Computer Engineering at Rice University in 2005 and was an Assistant then tenured Associate Professor of Electrical and Computer Engineering at Duke University from 2005 to 2013. She was an Associate Professor of Electrical and Computer Engineering, Harvey D. Spangler Faculty Scholar, and Fellow of the Wisconsin Institutes for Discovery at the University of Wisconsin-Madison from 2013 to 2018. Willett received the National Science Foundation CAREER Award in 2007, was a member of the DARPA Computer Science Study Group, and received an Air Force Office of Scientific Research Young Investigator Program award in 2010.