When calibration goes awry: hallucination in language models
Adam Tauman Kalai (OpenAI)
June 30 at 1:30pm
Abstract & Bio
Abstract:
We show that calibration, which is naturally encouraged by the loss minimized during pre-training of language models, leads to certain type of hallucinations. Moreover, the rate of hallucinations depends on the domain via the classic Good-Turing estimator. Interestingly, this estimate is small for domains like paper references which have been a notorious source of hallucinations. The analysis also suggests methods for mitigating hallucinations.
This is joint work with Santosh Vempala and was done while the speaker was at Microsoft Research New England.
Bio: Adam Tauman Kalai is a Research Scientist at OpenAI working on AI Safety and Ethics. Previously, he was a Senior Principal Researcher at Microsoft Research New England. He has worked in multiple fields, including Algorithms, Fairness, Machine Learning Theory, Game Theory, and Crowdsourcing. He received his BA from Harvard and PhD from Carnegie Mellon University. He has also served as an Assistant Professor at Georgia Tech and the Toyota Technological Institute at Chicago, and is a member of the science team of the whale-translation Project CETI. His honors include the Majulook prize, best paper awards, an NSF CAREER award, and an Alfred P. Sloan fellowship.
This is joint work with Santosh Vempala and was done while the speaker was at Microsoft Research New England.
Bio: Adam Tauman Kalai is a Research Scientist at OpenAI working on AI Safety and Ethics. Previously, he was a Senior Principal Researcher at Microsoft Research New England. He has worked in multiple fields, including Algorithms, Fairness, Machine Learning Theory, Game Theory, and Crowdsourcing. He received his BA from Harvard and PhD from Carnegie Mellon University. He has also served as an Assistant Professor at Georgia Tech and the Toyota Technological Institute at Chicago, and is a member of the science team of the whale-translation Project CETI. His honors include the Majulook prize, best paper awards, an NSF CAREER award, and an Alfred P. Sloan fellowship.
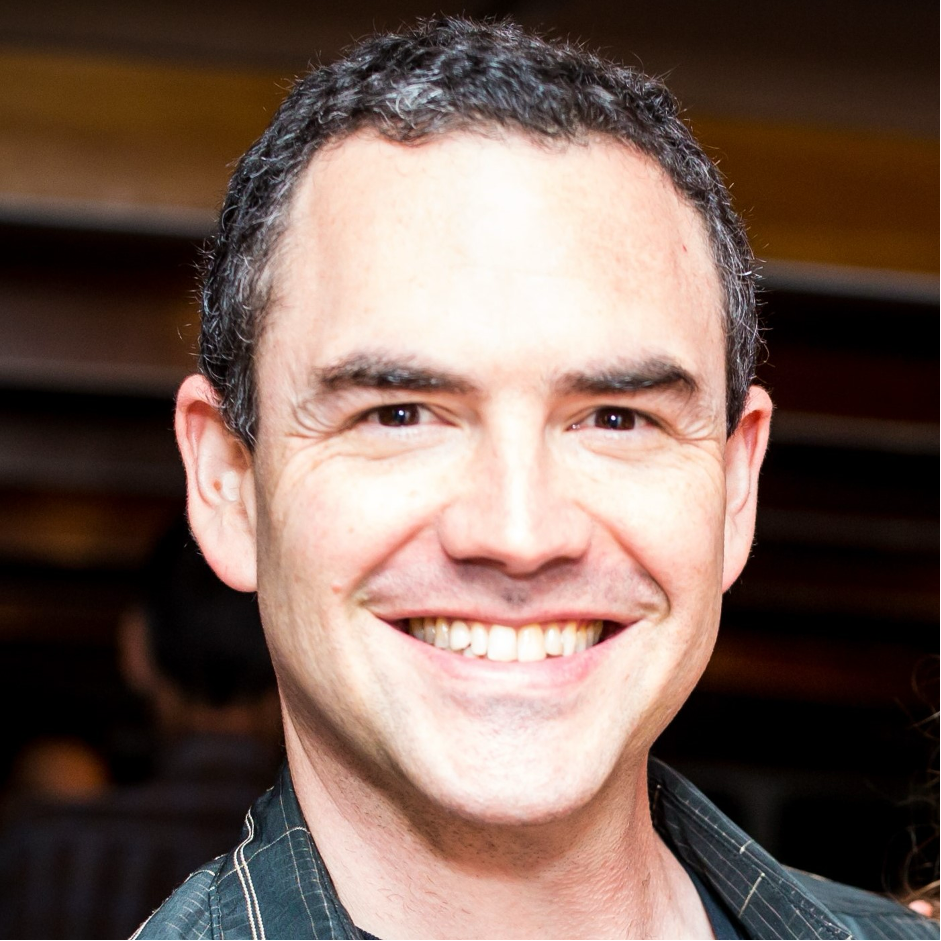